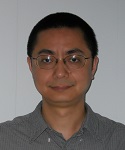
Prof. Yizhou Yu
University of Hong Kong, China
Title: Designing Deep Learning Algorithms in a Data Driven Way
Abstract:
In this talk, I use three examples to illustrate how datasets affect
the design of deep learning algorithms. For image classification
problems with a large label space, we designed hierarchical deep
convolutional neural networks capable of learning a category hierarchy
from data with uneven between-category distinctions. For fine-grained
classification problems with small training sets, we designed a novel
fine-tuning scheme called Selective Joint Fine-Tuning, which performs
multitask learning by sampling a subset of most relevant data from a
large source domain. For object detection or semantic segmentation
with image level labels only, we designed a weakly supervised learning
algorithm capable of inferring object level and pixel level labels
from image level labels for the training images.
Biography:
Yizhou Yu is a professor in the Department of Computer Science at the
University of Hong Kong. He was a professor at University of Illinois,
Urbana-Champaign (UIUC) from 2000 to 2010. He received his PhD degree
in computer science from University of California, Berkeley. Professor
Yu has made significant contributions to AI and visual computing,
including deep learning, computer vision, and computer graphics. He is
an ACM Distinguished Member and IEEE Fellow. His current research
interests also include biomedical data analysis, computational visual
media, and geometric processing. Professor Yu has served as an
associate editor of many international journals, including IET
Computer Vision, IEEE TVCG, The Visual Computer and International
Journal of Software and Informatics. He has also served on the program
committee of many leading international conferences, including
SIGGRAPH, SIGGRAPH Asia, and International Conference on Computer
Vision.