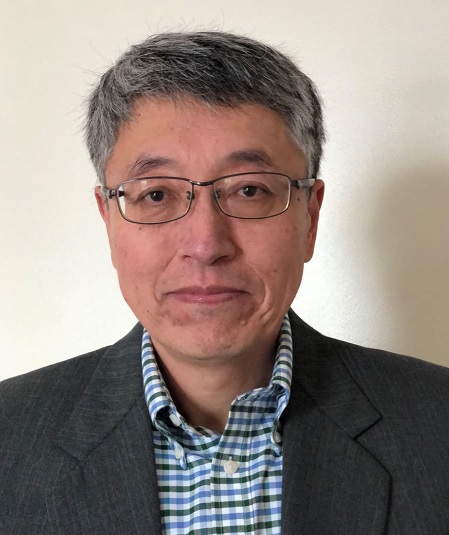
Prof. Guofan Shao
Purdue University, USA
Title: The Right Tool for the Right Job: Accuracy vs Efficacy
Abstract:
Image classification is one of the most important
tasks in many fields that involve image analysis. It is an ordinary approach to
evaluate classification performance by assessing the accuracy of classification
outcomes or maps. The quality of classification maps matters for downstream
applications, but the map accuracy values may not reflect the true performance of
image classification. This is because the existing accuracy measures have inherent
problems and can mislead the evaluation of classifier’s performance when class
size distributions are changed. There are two common misperceptions: accuracy
rates are assumed to comparable across maps and they reflect the discriminative
power of classifiers. This is particularly the case for classification with deep
learning because imbalanced datasets tend to undermine the performance of deep
learning models but favor some accuracy measures. Here we discuss and
demonstrate the use of image classification efficacy (ICE) to strengthen the
evaluation of image classification using deep learning. The introduction of ICE
helps clarify the distinctions between map’s accuracy assessment and classifier’s
performance evaluation. Such a differentiation is an important step toward
improved research on the evaluation and advancement of image classification in
the age of artificial intelligence.
Biography: