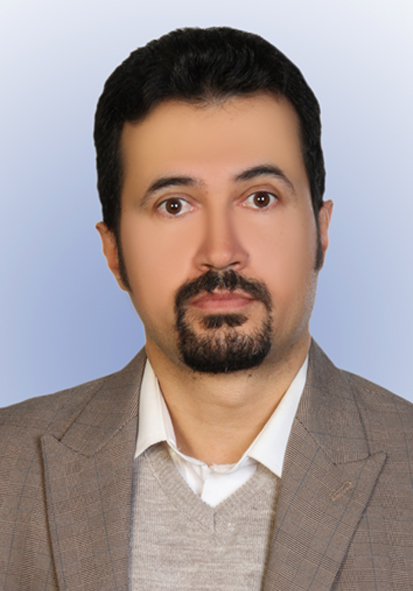
Associate Professor Mahdi Roozbeh
Semnan University, Iran
Title: Robust ridge estimator to combat outliers in high-dimensional semiparametric model using semidefinite programming VS support vector machines
Abstract:
In data science approaches, machine learning is a state of art class of robust techniques that can address many significant issues they might face in the modern world. The most popular of these techniques for classification is the support vector machine (SVM). Support vector regression (SVR) is a technique for developing a re-gression model that is an excellent addition to the machine learning family. High-dimensional (wide) data sets, where the number of observations is less than the number of parameters, have been the most challenging problem that may be faced in real-world applications, including econometric, social networks, psychological research, and the biosciences. The main difficulties in high-dimensional data sets are the estima-tion of the coefficients and model interpretation because classical methods, the same as the ordinary least-squares approach, which is the best approach if the classical as-sumptions, such as normality and independency of the errors, as well as a little or no multicollinearity between the explanatory variables, are met, are not applicable be-cause of a large number of predictor variables to the number of observations. SVR is one of the reliable and robust approaches to have a good fit with high accuracy ap-proach to model the high-dimensional data sets. In this research, a robust ridge esti-mator of the biased parameter are proposed based on the least trimmed squares (LTS) technique and its extensions using a semidefinite programming approach. It is shown that the proposed robust extended ridge estimator performs better than SVR in a real high-dimension dataset about the gene expression in the Riboflavin Vitamin B2 pro-duction data and then compare it with well-known method: least absolute shrinkage and selection operator method.
Keywords
High-dimensional data, Multicollinearity, Outlier, Penalized least trimmed squares, Ridge regression, Semiparametric model
Biography:
My name is Dr. Mahdi Roozbeh and I am an Associate Professor of Mathematics, Statistics, and Computer Science Department since 2018 at Semnan University. I graduated with a Ph.D. in Statistics from the Ferdowsi University of Mashhad in 2011, and I am an expert in regression modeling and analysis of high-dimensional data. I am winner of ISI (International Statistical Institute) Jan Tinbergen Award (International Statistical Study Fund Foundation for the young statisticians and the prize for excellence in statistics is awarded every two years) in 2011 (which hold every 2 years in the world) for the best paper award during the World Statistical Congress in Dublin, awards for Research Excellence at Semnan University in 2015; 2017; 2018 ;2020; 2021; 2023, Prof Behboodian Award and selected as the second Iranian young researcher in Statistics by Iranian Statistical Institute (2018), Elected for World Bank Trust Fund in the 62nd ISI WSC in Kuala Lumpur, 2019. My research focuses on high-dimensional deep learning, robust estimation and semiparametric regression modeling, and I am an expert in machine learning and invited as the keynote speaker in some international world congresses and published more than 50 papers indexed by ISI base and book chapters yet.
Reference
- https://scholar.google.com/citations?hl=en&user=KW9NMzoAAAAJ&view_op=list_works&sortby=pubdate
- https://www.scopus.com/authid/detail.uri?authorId=35590798700
- https://mahdiroozbeh.profile.semnan.ac.ir/#about_me
- https://www.researchgate.net/profile/Mahdi-Roozbeh
- https://www.webofscience.com/wos/author/record/1369569