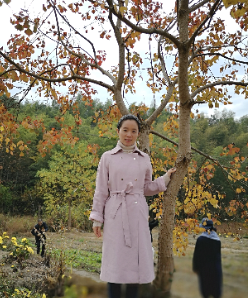
Prof. Le Sun
Nanjing University of Information Science and Technology, China
Title: Interpretable Classification for Medical Time Series Data
Abstract:
In this study, an innovative post-hoc explainability framework is proposed to systematically analyze the decision-making process of deep learning models in arrhythmia classification. By improving traditional feature importance analysis methods, the proposed framework can precisely identify key local segments in the signal and quantify their contribution to the model’s prediction results, thereby revealing the feature dependency relationships between different arrhythmia categories. The framework combines local morphological features with global temporal features, constructing a systematic explainability conceptual framework that includes morphological features such as P waves, QRS complexes, and T waves, as well as temporal features like heart rate variability, comprehensively elucidating the model’s feature selection mechanism. Experimental results based on the MIT-BIH arrhythmia database demonstrate that the optimized MobileNet model maintains high accuracy in classification tasks. By removing key segments and replacing them with random noise, feature importance was evaluated, and the results show a significant decrease in the model’s average confidence. Furthermore, the Pearson correlation coefficient consistency test validated the high consistency of our explainability conceptual framework in both global and local sample evaluations.
Biography:
Dr. Le Sun is a Professor in the School of Computer and Software, Nanjing University of Information Science and Technology, China. She received her PhD degree in 2016, from Victoria University, Australia, and received the Chancellor-citation Best PhD Award. In 2018, she was a visiting scholar of Michigan State University. She has published over 50 papers on high quality conferences and journals, including CCF-A, B and C journal papers. Her research interests include Medical data stream mining, services computing, and fog computing.