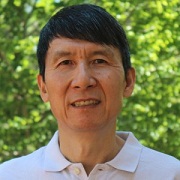
Prof. Jin Wang
Northern Arizona University, USA
Title: Efficiency and Robustness of Depth-Based Trimmed Means and Trimmed Scatter Matrices
Abstract:
Two important criteria to evaluate the performance of an estimator or a statistic are efficiency and robustness. This research focuses on the efficiency and robustness properties of the depth-based trimmed means and trimmed scatter matrices of Wang (2025). Specifically, we compute the asymptotic relative efficiencies of the trimmed means with respect to the sample mean vector and the asymptotic relative efficiencies of the trimmed scatter matrices with respect to the sample covariance matrix for various multivariate distributions. The local robustness of the trimmed means and trimmed scatter matrices is explored through influence function and the global robustness through finite-sample breakdown point. The results show that the trimmed means and trimmed scatter matrices are not only highly efficient but also exceptionally robust, making them highly competitive estimators for multivariate location and scatter.
Biography:
Jin Wang is a professor of statistics at Northern Arizona University (NAU), USA. His main research areas include nonparametric multivariate analysis, biostatistics, reliability analysis, probabilistic risk analysis, change-point problems, and asymptotic theory. His contributions to nonparametric multivariate statistics include a nonparametric multivariate kurtosis measure (Wang and Serfling, 2005), a family of kurtosis orderings for multivariate distributions (Wang, 2009), a generalized spread function and a generalized multivariate kurtosis ordering (Wang and Zhou, 2012), a graphical method to compare spread and kurtosis of two multivariate data sets and a new graphical method to assess multivariate normality (Wang, 2019), and so on. Besides theoretical research, Wang is also interested in applications of statistics in various fields. He worked in industry for eight years (1991-1999) and worked on several health-related projects at NAU.