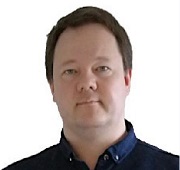
Prof. Pavel Loskot
ZJU-UIUC Institute, China
Title: Exploiting Approximations in Signal Processing
Abstract:
The approximations are a powerful tool to enable numerical and mathematical tractability of many signal processing tasks. They are usually defined as smooth parameterized functions, so that their parameters can be determined to minimize the approximation error. In this talk, I will outline several approximation strategies, which are used to perform Bayesian inferences involving intractable multi-dimensional distributions, modeling multi-dimensional functions as Gaussian processes in Bayesian optimizations, designing non-linear filters using linearizations, performing linear regression in multiple dimensions, and even computing the integrals and solving differential equations using various approximations. The goal is to identify the common principles underlying the use of approximations in solving different signal processing problems.
Biography:
Nearly 30 years of experience in mathematical modeling, signal processing and data mining related to various engineering as well as biological systems. As Principal Investigator and Co-Investigator, involved in numerous academic and industrial collaborative projects. Over 10 years of direct experience in cross-disciplinary collaborations at intersection of engineering, mathematics, biology, and economics in the areas spanning rural Internet, IoT, wireless sensor networks, computational molecular biology, renewable energy and air transport management. Expert level knowledge of designing and implementing statistical and digital signal processing algorithms and methods. Solid background in applied probability and statistics. Avid Linux user and programmer since 1996. The current research focus on adopting the methods and strategies from telecommunication engineering and computer science to improve the efficiency of system modeling and analysis. The research topics of particular interest include designing computer experiments, probabilistic modeling, automated hypothesis discovery, signals processing with incomplete and/or constrained knowledge, signal representations for efficient statistical learning, and distributed signal processing.