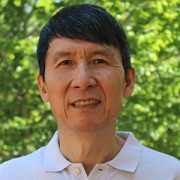
Prof. Jin Wang
Northern Arizona University, USA
Title: On General Depth-Based Trimmed Means and Trimmed Covariance Matrices
Abstract:
Multivariate descriptive
measures for location and scatter are the foundation of multivariate statistics. Many
multivariate statistical methods are based on them. The classical measures of
these concepts are moment-based and are estimated by the sample mean vector and the sample covariance
matrix. However, these estimators are not robust and are extremely sensitive to
outliers. To eliminate or reduce the effects of outliers,
we propose general depth-based trimmed means and trimmed covariance matrices.
The basic properties of the trimmed means and trimmed covariance matrices are studied.
It is shown that these new estimators are not only robust but also efficient, and
one can balance robustness and efficiency by trimming percentage in practical
applications. Under some regularity conditions, both the trimmed means and trimmed
covariance matrices are strongly consistent and asymptotically normal.
Biography:
Jin
Wang is a professor of statistics at Northern Arizona University (NAU), USA. His main research areas include
nonparametric multivariate analysis, biostatistics, reliability analysis,
probabilistic risk analysis, change-point problems, and asymptotic
theory. His contributions to nonparametric multivariate statistics include a
nonparametric multivariate kurtosis measure (Wang and Serfling, 2005), a family
of kurtosis orderings for multivariate distributions (Wang, 2009), a
generalized spread function and a generalized multivariate kurtosis ordering
(Wang and Zhou, 2012), a graphical method to compare spread and kurtosis of two
multivariate data sets and a new graphical method to assess multivariate
normality (Wang, 2019), and so on. Besides theoretical research, Wang is also
interested in applications of statistics in various fields. He worked in
industry for eight years (1991-1999) and worked on several health-related
projects at NAU.