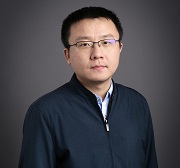
Associate Professor Jie Chen
Xi'an Jiaotong-Liverpool University, China
Title: Machine Learning-based Generalized Multiscale Finite Element Method and its Application in Reservoir Simulation
Abstract:
In multiscale modeling of subsurface fluid flow in heterogeneous porous media, standard polynomial basis functions are replaced by multiscale basis functions, which are used to predict pressure distribution. To produce such functions in the mixed Generalized Multiscale Finite Element Method (GMsFEM), a number of Partial Differential Equations (PDEs) must be solved, leading to significant computational overhead. The main objective of the work presented in this talk was to investigate the efficiency of Deep Learning (DL) models in reconstructing the multiscale basis functions of the mixed GMsFEM. To achieve this, four standard models named SkiplessCNN models were first developed to predict four different multiscale basis functions (Basis 2 to 5). These predictions were based on two distinct datasets (initial and extended) generated, with the permeability field being the sole input. Subsequently, focusing on the extended dataset, three distinct skip connection schemes (FirstSkip, MidSkip, and DualSkip) were incorporated into the SkiplessCNN architecture. Following this developed four models - SkiplessCNN, FirstSkipCNN, MidSkipCNN, and DualSkipCNN - were separately combined using linear regression and ridge regression within the framework of Deep Ensemble Learning (DEL). Furthermore, the reliability of the Dual-SkipCNN model was examined using Monte Carlo (MC) dropout. Ultimately, two Fourier Neural Operator (FNO) models, operating on infinite-dimensional spaces, were developed based on a new dataset for directly predicting pressure distribution. Based on the results, sufficient data for the validation and testing subsets could help decrease overfitting. Additionally, all three skip connections were found to be effective in enhancing the performance of SkiplessCNN, with DualSkip being the most effective among them. As evaluated on the testing subset, the combined models using linear regression and ridge regression meaningfully outperformed the individual models for all basis functions. The results also confirmed the robustness of MC dropout for DualSkipCNN in terms of epistemic uncertainty. Regarding the FNO models, it was discovered that the inclusion of an MLP in the original Fourier layers significantly improved the prediction performance on the testing subset. Looking at this work as an image (matrix)-to-image (matrix) problem, the developed data-driven models through various techniques could potentially find applications beyond reservoir engineering.
Biography:
Associate Professor Jie Chen obtained his Bachelor's and Master's degrees from the Department of Mathematics at Nanjing University in 2004 and 2007, respectively. He completed his Ph.D. at Nanyang Technological University in 2011. From August 2011 to October 2012, he conducted postdoctoral research at the Hong Kong University of Science and Technology, followed by another postdoctoral research position at King Saud University from November 2012 to March 2013. In April 2013, he joined the School of Mathematics and Statistics at Xi'an Jiaotong University, and in August 2019, he joined Xi'an Jiaotong-Liverpool University. His research interests include finite element methods, computational fluid dynamics, and reservoir simulation. He has published over thirty papers in prestigious international journals such as Mathematics of Computation, Journal of Computational Physics, and International Journal for Numerical Methods in Engineering. Additionally, he has led and participated in over ten research projects, including general programs, youth funds, postdoctoral special funds, and CMG funds. He serves as a reviewer for more than 20 international journals, including the Journal of Computational Physics, Computer Methods in Applied Mechanics and Engineering, Water Resources Research, and the International Journal for Numerical Methods in Engineering. Furthermore, he is an expert reviewer for the evaluation of doctoral theses appointed by the Ministry of Education. In 2019, Jie Chen attended the 8th Chinese Mathematicians Conference and delivered a 45-minute invited presentation.